How to Find the Best Deployable Edge Computing Platforms
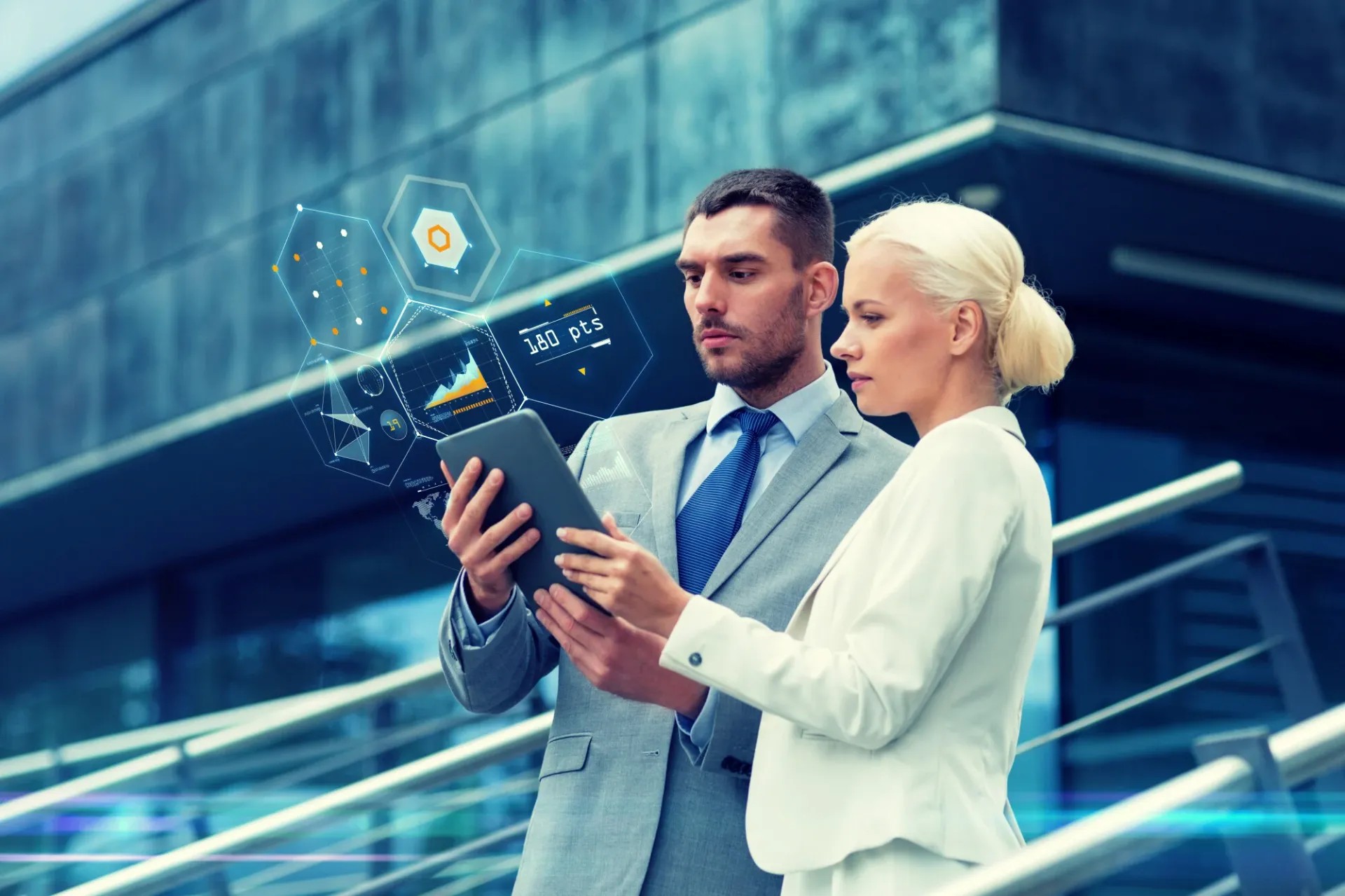
To make the most of deployable edge computing capabilities in an open intelligence ecosystem for the purpose of gathering, aggregating, and analyzing multisource data from all corners of the world, you must ensure you have access to the necessary tools and platforms.
In today's data-driven world, the ability to process and derive insights from massive amounts of data generated at the edge is of paramount importance. This is the context in which deployable edge computing platforms become essential, and discovering the most suitable one that matches your requirements can have a substantial influence on your data analysis and decision-making procedures.
PySpark, a Python library designed for Spark, stands out as a potent tool in this realm, empowering you to effectively handle and scrutinize extensive datasets. Utilizing the functionalities of PySpark opens up avenues for performing sophisticated data processing operations, encompassing intricate joins facilitated by the PySpark join function, thereby significantly elevating your data analysis proficiencies. However, the effectiveness of your PySpark operations can be further enhanced by optimizing your Spark configuration to suit the specific requirements of your deployment.
Java Spark emerges as an additional pivotal factor to contemplate, owing to its capability to enable the construction of resilient and scalable applications customized for deployable edge computing platforms. Furthermore, a comprehensive understanding of knowledge graphs can prove invaluable in deploying edge computing platforms effectively. These graphical representations that depict interconnected nodes of information can aid you in proficiently modeling data and establishing associations among various data elements.
When it comes to predictive modeling, having the right set of tools is essential. The role played by data modeling tools is of immense consequence, as they are instrumental in crafting precise and effective models that can underlie insightful predictions and decisions. Moreover, the construction of a well-structured machine learning pipeline is indispensable for the triumph of your deployable edge computing platform. This pipeline directs the trajectory of data from its rudimentary format to a polished state, enabling it to navigate through assorted stages of processing, analysis, and modeling, ultimately culminating in the derivation of significant outcomes.
Furthermore, the choice of an appropriate ETL (Extract, Transform, Load) tool holds immense significance in ensuring efficient data management within your deployable edge computing platform. ETL tools play a pivotal role in enabling the uninterrupted transition of data across diverse junctures of your data processing pipeline, thereby ensuring precise and efficient extraction, transformation, and loading of data.
In the domain of computing, the advent of cloud services has brought about a revolution in the manner in which data is managed, processed, and scrutinized. Embedded within cloud computing, platform as a service offers furnish developers and data scientists with an all-encompassing milieu to construct, launch, and oversee applications and data analytics pipelines, all devoid of the intricacies associated with infrastructure management. By opting for PaaS solutions, you can focus on the core aspects of your deployable edge computing platform, such as data analysis and application development, while the underlying infrastructure, including hardware and networking, is taken care of by the cloud service provider.
You can get more enlightened on this topic by reading here: https://en.wikipedia.org/wiki/Analytics.